Across industries, the demand for AI processing is growing exponentially, primarily driven by the increasing complexity of new generative AI models, which feature an exponential growth in the number of parameters and the size of training datasets. OpenNebula Systems has just released an Industry Report that estimates the expected growth in computing needs for Generative AI training and inference from 2024 up until 2030. The main challenges in AI development analyzed in this Industry Report include data availability, scalability limitations of centralized systems, power constraints, and challenges in accelerator manufacturing.
This study assumes that AI training and inference have distinct execution profiles, each requiring different infrastructure architectures to meet their specific needs. While training will be typically carried out in the cloud or on supercomputers, inference will primarily occur on-demand on edge computing platforms, often managed by telecom operators.
In 2024, the performance demand for a single AI training run has been estimated to reach 1 exaflop/s. As of June 2024, the Frontier Supercomputer in the United States remains the leading HPC system globally, achieving a peak performance of 1.7 exaflop/s. In September 2024, Oracle announced the world’s largest, first AI zettascale supercomputer in the cloud. This system will feature 131,072 GPUs, which is more than three times the number of GPUs in the Frontier Supercomputer, and will be able to scale up to 2.4 zettaflop/s. Oracle didn’t confirm when its Blackwell-powered service will come online, considering that the chips it requires are currently still being manufactured.
AI Training in 2030 will require systems able to deliver between 100 and 10,000 exaflop/s. The performance of the top TOP500 supercomputer has doubled every two years over the past decade. By 2030, the top HPC and GPU-accelerated cloud systems in the US are expected to deliver up to 20 exaflop/s and 200 exaflop/s, respectively, while in the EU, they are projected to reach 5 exaflop/s and 20 exaflop/s. This represents a roughly 10x increase in performance. In the peak scenario, the US and EU are expected to present a x100 and x1000 performance gap, respectively.
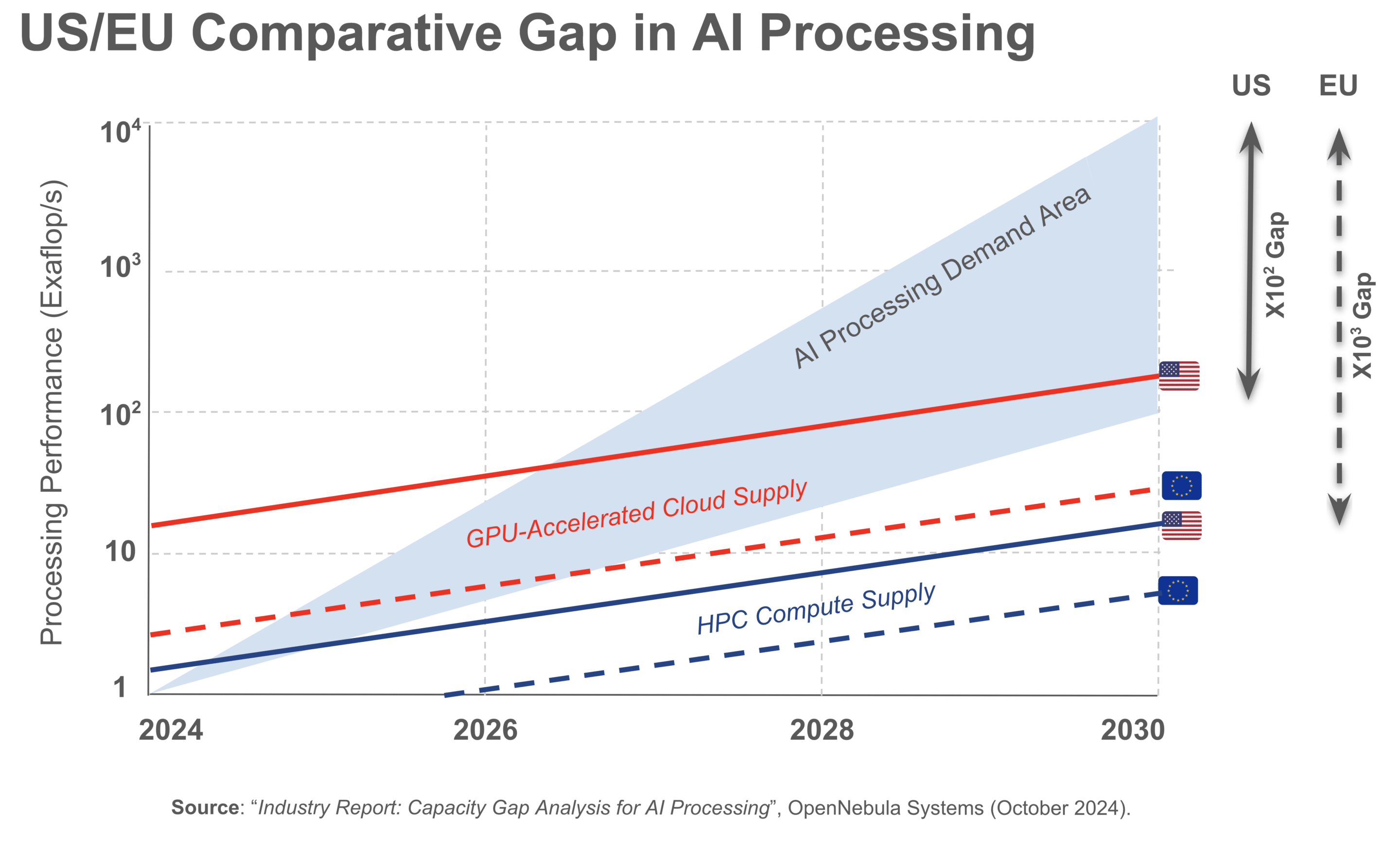
By 2030, the performance demand for a single AI training run is expected to reach up to 10 zettaflop/s. The primary bottlenecks in achieving this performance within a single HPC or cloud system include scalability limitations of centralized architectures and power constraints. These challenges highlight the importance of developing more efficient AI algorithms, advancing both technological and architectural solutions to improve HPC performance, and prioritizing research into more efficient hardware, optimized software programming, and energy-efficient data centers, among other innovations.
From a strategic perspective, the most effective way to meet future AI processing needs is through the development of new distributed and decentralized systems. Leveraging a continuum of HPC, cloud, and edge resources will be crucial for addressing the intensive processing demands of AI training and the low-latency requirements of AI inference. This includes:
- Developing new open and decentralized AI models and applications that spread workloads across multiple data centers, regardless of their physical proximity, to meet the future challenges of AI processing, while enabling a more secure, resilient, and potentially fairer AI ecosystem.
- Accelerating the creation of a high-performance, distributed cloud-edge continuum across, that will be able to meet the growing demands of both AI and ultra-low-latency applications in the future.
- Developing dedicated low-latency networks to interconnect HPC systems is essential for executing tightly-coupled AI training models.
As part of the new €3B IPCEI-CIS initiative, OpenNebula Systems, in collaboration with other leading cloud computing players, is developing a management and orchestration platform for creating cloud-edge environments that meet the needs of AI processing workflows and the high-performance and low-latency requirements of their components.
0 Comments